Usage¶
Installation¶
To use SwingTrend
, first install it using pip:
$ pip install swingtrend
Swing class requires atleast 40 candles (Recommended 60 candles) to get an accurate reading of the trend.
Examples¶
For conciseness, only the relevant bits of code have been shown.
Basic example¶
from swingtrend import Swing
swing = Swing(retrace_threshold_pct=5)
swing.run(sym="HDFCBANK", df=df.iloc[-60:])
print(f"{swing.symbol} - {swing.trend}")
if swing.trend == "UP":
print(f"SPH: {swing.sph}, {swing.sph_dt:%d %b %Y}")
print(f"CoCh: {swing.coc}, {swing.coc_dt:%d %b %Y}")
elif swing.trend == "DOWN":
print(f"SPL: {swing.spl}, {swing.spl_dt:%d %b %Y}")
print(f"CoCh: {swing.coc}, {swing.coc_dt:%d %b %Y}")
swing.is_sideways # bool True or False
# Are enough candles present to accurately determine trend?
swing.is_trend_stable # bool True or False
swing.reset()
Screening stocks¶
for sym in watchlist:
swing.run(sym=sym.upper(), df=df.iloc[-60:])
if swing.trend == "UP" and swing.bars_since > 4 and swing.bars_since < 15:
# Stocks in uptrend with a pullback between 4 and 15 bars.
print(sym)
swing.reset() # Dont forget to reset after each iteration.
Attaching callback functions¶
Here we look for stocks which have reversed to uptrend or broken above the SPH.
The stocks are collected into a list and printed at the end.
breakout_lst = []
reversal_lst = []
# The two functions below will be attached to the Swing class
def bos(swing: Swing, date, close, breakout_level):
if date != swing.df.index[-1]:
# We only want stocks for today and not previous dates.
return
if swing.trend == "UP" and swing.is_trend_stable:
breakout_lst.append(swing.symbol)
print(
f"{date:%d %b %Y}: {swing.symbol} break @ {breakout_level} with close @ {close}"
)
def reversal(swing: Swing, date, close, reversal_level):
if date != swing.df.index[-1]:
return
# Trend was down and now reversed to UP
if swing.trend == "UP" and swing.is_trend_stable:
breakout_lst.append(swing.symbol)
print(
f"{date:%d %b %Y}: {swing.symbol} reversed @ {reversal_level} with close @ {close}"
)
swing = Swing()
# Attach the functions to Swing class
swing.on_breakout = bos
swing.on_reversal = reversal
for sym in watchlist:
swing.run(sym=sym.upper(), df=df.iloc[-60:])
swing.reset()
if breakout_lst:
print("Breakouts", breakout_lst)
if reversal_lst:
print("Reversals", reversal_lst)
Plot with mplfinance¶
import mplfinance as mpf
from swingtrend import Swing
swing = Swing(retrace_threshold_pct=8)
# add `plot_lines=True`
# here we pass additional candles since it takes 40 candles to confirm the trend.
swing.run(sym, df.iloc[-160:], plot_lines=True)
# `swing.plot_lines` provides the line coordinates
# `swing.plot_colors` provides the line colors
# Add the lines and colors to alines
mpf.plot(
df,
title=f"{sym.upper()} {swing.trend}",
type="candle",
style="tradingview",
scale_padding=dict(left=0.05, right=0.6, top=0.35, bottom=0.7),
alines=dict(
linewidths=0.8,
alpha=0.7,
colors=swing.plot_colors,
alines=swing.plot_lines,
),
)
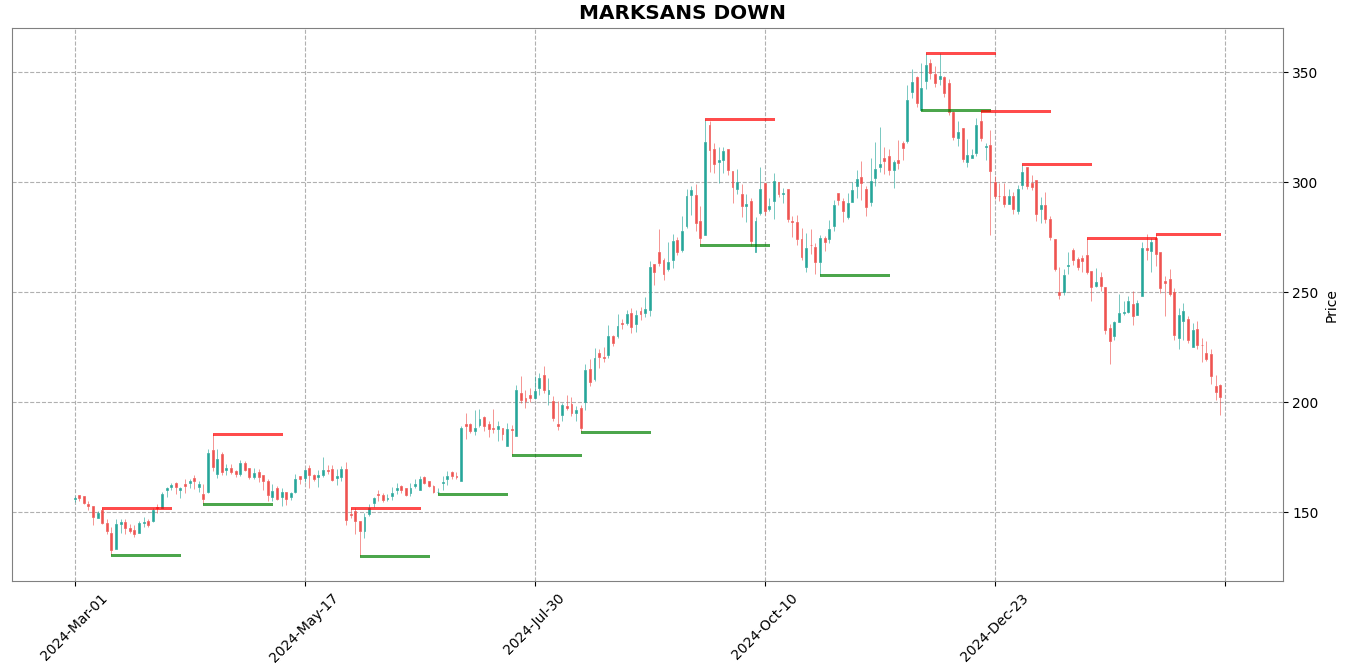
In the chart, green lines indicate the CoCh levels in uptrend. Red line represent CoCh levels in downtrend.¶
Using Swing.identify
¶
Pandas is not a requirement. You can provide OHLC data from any source to Swing.identify
.
You can also pass OHLC data generated from websocket feeds for real-time trend identification.
ohlc_tuple = (
(datetime(2024, 1, 1), 100, 90, 93),
(datetime(2024, 1, 2), 95, 85, 88),
(datetime(2024, 1, 3), 90, 80, 83),
(datetime(2024, 1, 4), 85, 75, 78),
)
swing = Swing()
for tup in ohlc_tuple:
swing.identify(*tup)
Debug Mode¶
Debug mode is useful when trying to understand the program. Have a chart in front and read the logs.
import logging
from swingtrend import Swing
# Make sure to set basicConfig for logging
logging.basicConfig(format="%(levelname)s: %(message)s", level=logging.WARNING)
swing = Swing(debug=True)
# DEBUG: 2024-12-09 00:00:00: First Candle: High 377.45 Low: 370.1
# DEBUG: 2024-12-10 00:00:00: Start Trend: UP High: 383.4
# DEBUG: 2024-12-11 00:00:00: New High: 388.65
# DEBUG: 2024-12-12 00:00:00: New High: 391.5
# DEBUG: 2024-12-13 00:00:00: Swing High - UP SPH: 391.5 CoCh: 370.1
# DEBUG: 2024-12-17 00:00:00: Reversal DOWN Low: 363.25 CoCh: 391.5
# DEBUG: 2024-12-18 00:00:00: New Low: 362.3
# DEBUG: 2024-12-19 00:00:00: New Low: 358.6
# DEBUG: 2024-12-20 00:00:00: New Low: 355.2
# DEBUG: 2024-12-23 00:00:00: New Low: 346.45
# DEBUG: 2024-12-24 00:00:00: Swing Low - DOWN SPL: 346.45 CoCh: 391.5
Adding a series to Pandas DataFrame¶
Set add_series
argument in swing.run to True. This is useful for backtesting historical data.
The first 40 candles will be None, since the trend is not fully confirmed till then.
swing = Swing()
swing.run(sym=sym.upper(), df=df, add_series=True)
print(df)
# Open High Low Close Volume TREND IS_SIDEWAYS
# Date
# 2024-07-15 250.81 255.00 242.76 252.98 2601155 None None
# 2024-07-16 252.80 256.50 247.00 254.01 3210967 None None
# 2024-07-18 250.00 258.00 244.00 245.16 3355053 None None
# 2024-07-19 248.00 258.59 241.60 244.69 8460052 None None
# 2024-07-22 244.50 249.39 240.81 244.57 2066923 None None
# ... ... ... ... ... ... ... ...
# 2025-02-24 364.00 364.00 355.20 360.25 1305661 0 0
# 2025-02-25 358.00 365.35 352.00 354.05 2113683 0 0
# 2025-02-27 357.00 362.95 347.60 356.75 1797225 0 1
# 2025-02-28 353.00 355.40 340.00 346.00 2773439 0 1
# 2025-03-03 342.50 356.95 327.85 352.10 2583375 0 1
#
# [160 rows x 7 columns]